MDLMA - Multi-task Deep Learning for Large-scale Multimodal Biomedical Image Analysis
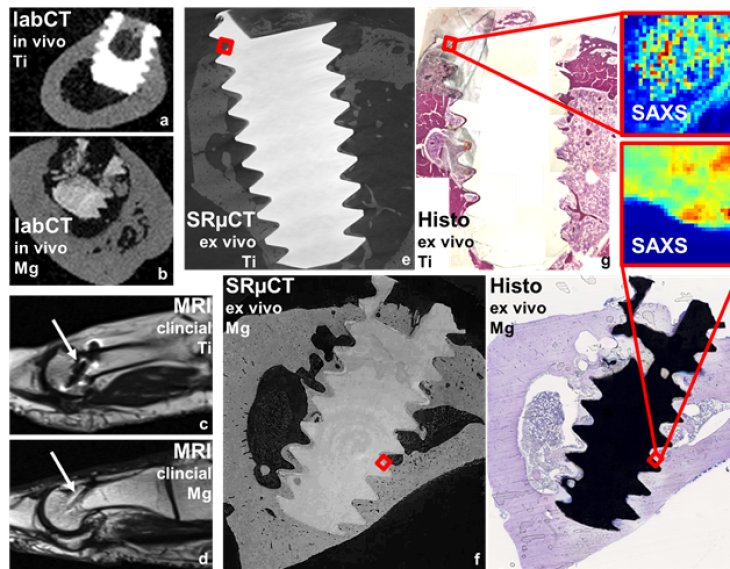
Imaging modalities for the characterization of biodegradable Mg-based implants in bone and the multimodal registration of which
MDLMA, acronym for ‘Multi-task Deep Learning for Large-scale Multimodal Biomedical Image Analysis’, is a joint research project of the Helmholtz-Zentrum Hereon, the Deutsche Elektronen-Synchrotron DESY, the University of Lübeck (UzL) and the company Syntellix AG. It is funded by the Federal Ministry of Education and Research (BMBF), grant number 031L0202A. The project is lead and coordinated by Prof. Dr. Regine Willumeit-Römer and Dr. Julian Moosmann.
In order to optimise biodegradable Mg-based implants with respect to their mechanical, biological and degradation properties, to understand the mechanisms governing the interactions between microstructure, mechanical properties, biology and degradation, and to tailor implants for specific applications, large amounts of multimodal biomedical image data have to be analyzed. Modalities include laboratory X-ray computed tomography (CT), synchrotron radiation micro-computed tomography (SRμCT), magnetic resonance imaging (MRI), small angle X-ray scattering (SAXS) and histology. Recurrent image analysis tasks considered within this project are registration, segmentation/classification, and image enhancement (e.g. artifact or noise reduction) using deep learning (DL) approaches. Further, we will devise new multi-task DL methods that are able to integrally combine different complementary tasks and transfer knowledge across individual analysis tasks. In order to facilitate the application of these multi-task solutions for other domains, modalities and tasks, a unified framework will be developed that enables a quick and efficient implementation and application of data analysis tasks.
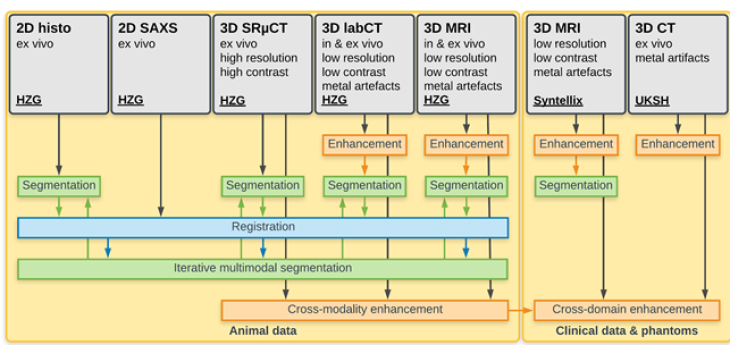
Schematic overview of imaging modalities, data sets and analysis tasks